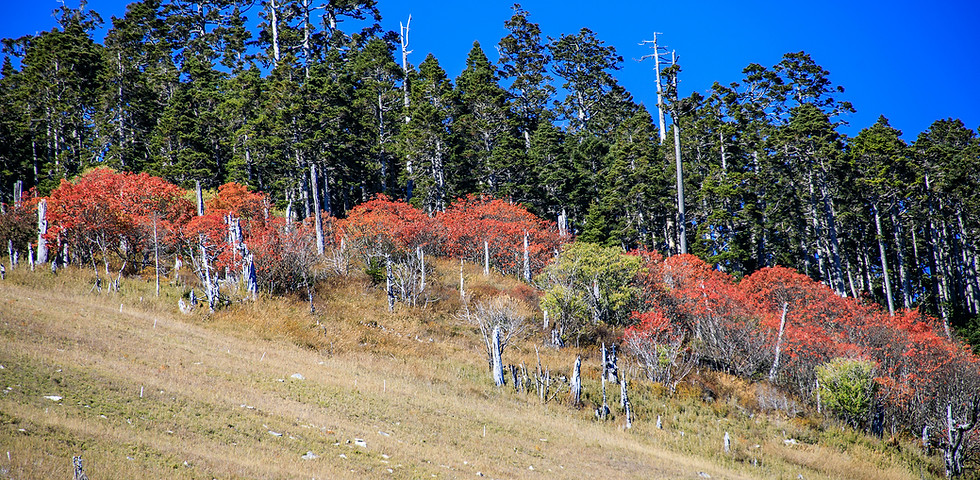
1301 Probability
-
Course Time: Mon 09:10-12:00
-
Classroom: AT242
-
Course Outlines: 1. Experiments, Models and Probabilities; 2. Sequential Experiments; 3. Discrete Random Variables; 4. Continuous Random Variables. 5. Multiple Random Variables; 6. Probability Models of Derived Random Variables; 7. Conditional Probability Models; 8. Random Vectors; 9. Sums of Random Variables; 10. The Sample Mean; 11. Hypothesis Testing; 12. Estimation of a Random Variable; 13. Stochastic Processes.
-
Textbook: Probability and Stochastic Processes: A Friendly Introduction for Electrical and Computer Engineers ", the 3rd Edition, by Roy D. Yates and David J. Goodman (John Wiley & Sons), 2015.
-
Reference Books:
-
Introduction to Statistical Pattern Recognition, by Keinosuke Fukunaga, 2nd Edition, Academic Press, 1990.
-
Introduction to Probability and Statistics: for Engineering and the Computing Sciences, by J. Susan Milton, Jesse C. Arnold, Liu Kwong Ip, the McGraw Hill Companies.
-
R in ACTION: Data analysis and graphics with R, by Kabacoff, Robert I., Manning Publications, 2015.
-
-
Lecture Notes: Probability00
-
Grade: 成績 (updated at 06/27)
-
News:
-
HW1: (a) Programming: quiz 1.6 (b) 1.1.2 and 1.3.1
-
HW2: (a) Programming: quiz 2.4 (b) 2.1.10 and 2.2.10
-
3/25 小考, Range: chapter 1 & chapter 2.
-
HW3: (a) Programming: quiz 3.9 (b) 3.2.10, 3.3.13 and 3.5.10.
-
4/22 09:30 期中考,Ranges: chapter 1, chapter 2 and chapter 3.
-
5/20 小考, Range: chapter 4.
-
HW4: (a)Programming: Generate 50 samples with the distribution N(60,10), and compute the mean, std, and median. (b) 4.6.15, and 4.7.1.
-
HW5: Problems 5.5.6 and 5.7.3
-
6/17 09:30 期末考,Ranges: chapter 4, chapter 5 and chapter 8.
-
期末考與學期成績已公佈,有疑問者請於6/27上午0900-1200與助教聯絡,學期成績有疑問者,請於6/27上午0900-1200與我聯絡,逾時不後。
-
6638 Pattern Recognition
-
Course Time: Tue 13:10-16:00
-
Classroom: AT338
-
Course Outlines: 1.Introduction; 2.Bayes Decision Theory; 3.Maximum-Likelihood and Bayesian Parameter Estimation; 4.Nonparametric Techniques; 5.Linear Discriminant Functions; 6.Multilayer Neural Networks; 7. Deep Learning - Convolutional Neural Networks; 8. Nonparametric Methods; 9. Unsupervised Learning and Clustering.
-
Textbook: "Pattern Classification", by Richard O. Duda, Peter E. Hart and David G. Stork, John Wiley & Sons, 2nd edition, 2001.
-
Reference Books:
-
Introduction to Statistical Pattern Recognition, by Keinosuke Fukunaga, 2nd Edition, Academic Press, 1990.
-
Neural Networks and Learning Machines, 3rd Edition, Simon O. Haykin, McMaster University, Ontario Canada, Pearson, 2009.
-
Statistical Pattern Recognition, by Andrew Webb, Second Edition, John Wiley & Sons, 2002.
-
R in ACTION: Data analysis and graphics with R, by Kabacoff, Robert I., Manning Publications, 2015.
-
-
Lecture Notes: PR00, PR01, MathFoundation, RandomVectors, PR02a , PR02b , Parametric Classifiers, PR06 , PR10, Deep learning
-
Grade: 成績 (updated at 06/25)
-
News:
-
HW1: Design the Maximum A Posterior (MAP) classifier for the UCI-Sonar dataset (2 classes, 60 features) if 75% of the data are used in the training phase, and the rest are used to evaluate. The deadline is 23th April.
-
HW2: (a) Compute Bhattacharyya distance for the UCI-Sonar dataset; (b) Compute Bhattacharyya and Chernoff error bound for the UCI-Sonar dataset. And have a discussion with HW1. The deadline is 14th May.
-
HW3: Single layer perceptron for the UCI-Sonar dataset. Please compare the result with HW1. The deadline is 28th May.
-
5/14 課程因到成大開會暫停一次
-
Final Project: 期末專題每組2-4人,分組名單與Proposal (one page) 請於5/28交,題目內容須用到本課程所涵蓋的分類器(classifiers)。表現優良的組別,推薦參加6/14下午1400-1600舉行的「興創跨領域期末成果展」,地點綜合大樓109翻轉教室。6/11 and 18 舉行期末報告。
-
HW4: Self-Organizing Map Network for the IRIS and Sonar datasets. The deadline is 10th June.
-
6/4 13:30 舉行期末考。
-
作業繳交方式:內容為主要演算法與程式片斷,包含測試資料與結果,以及簡短的討論或結論等。請把書面報告電子檔,程式及相關測試資料先以Winzip或WinRAR壓縮,並以學號為其檔名,上傳到以下指定的FTP網站:IP:140.120.182.124 , Port: 1996, Username: prstudent, Password: pr19
-
期末考與學期成績已公佈,作業有疑問者請於6/27上午0900-1200與助教聯絡,期末考與學期成績有疑問者,請於6/27上午0900-1200與我聯絡,逾時不後。
-
3535 Digital Photography and Image Processing (數位攝影與影像處理)
-
Course Time: Tue 18:20-20:00
-
Classroom: L216 (水保系二館二樓 電腦教室)
-
Course Outlines: 1.認識數位單眼相機DSLR; 2 基礎攝影理論介紹, 3.數位暗房RAW檔的處理,以Lightroom為例; 4.Photoshop 基礎影像處理; 5.Photoshop 進階影像處理,含筆刷工具,液化工具等; 6. Photoshop 高階影像處理,含物件去背,圖層使用,影像合成,批次處理等; 7. 高動態範圍(HDR)影像製作與處理; 8. 寬景照(Panorama) 影像製作與處理; 9. 閃光燈基本理論與進階使用技巧; 10. 數位影像多媒體光碟製作; 11. 影片剪輯與後製處理,以會聲會影(Video Studio)為例; 12. 縮時攝影。
-
Textbook: 正確學會數位攝影的16堂課,施威銘/主編 ,旗標出版公司
-
Reference Book:
-
抓住你的Photoshop CS6,施威銘研究室著,旗標出版股份有限公司 (ISBN:9789863120735 )
-
Lightroom魅力人像修圖,侯俊耀,碁峯出版社
-
會聲會影X5影片剪輯,旗標出版股份有限公司
-
-
Lecture Notes: DPIP,攝影理論簡介,閃光燈
-
Grade: 成績 (updated at 06/24)
-
News:
-
HW1: 夜曝(長曝光)練習,其中一張照片的快門須超過30秒。
-
HW2: 權衡式測光 vs. 重點測光
-
HW3: 以黑卡拍攝高反差場景
-
4/23 18:30-20:00 期中考,地點:綜合大樓Y312教室
-
HW4: Lightroom三種全域影像處理功能,需要包含白平衡與相機變形校正。
-
HW5: Lightroom全域影像處理HSL與黑白功能。
-
6/4 晚上20:20 舉行小考,範圍:色彩空間與Lightroom。
-
HW6: Lightroom三種局部影像處理功能,需要調整筆刷與漸層濾鏡。
-
6/18 18:30-20:00 期末考,地點:綜合大樓Y313教室
-
期末考與學期成績已公佈,有疑問者請於6/27上午0900-1200與助教聯絡,學期成績有疑問者,請於6/27上午0900-1200與我聯絡,逾時不後。
-
1505 Business Computer Programming (商業程式設計)
-
Course Time: Thu 18:20-20:00
-
Course Outlines: 學習R語言於統計與資料分析的應用,R語言程式設計,R語言繪圖與報表,R語言在人工智慧等的應用。內容包含有:1. RStudio 開發環境的建置與介紹; 2. 變數型態、向量運算、函數的使用; 3. 各種資料的讀取與匯入; 4. 直覺、吸睛的繪圖技巧; 5. 原始資料的整併和取樣; 6. 字串的處理與運算; 7. 迴圈、向量等群組資料的操作; 8. 報表、簡報和網頁呈現的技巧; 9. 各種統計、迴歸資料模型的應用; 10. R 軟體於深度學習(CNN)的應用
-
Textbook: R 軟體 資料分析基礎與應用 第二版 2019 (R for Everyone: Advanced Analytics and Graphics),旗標出版公司
-
Reference Book: R in Action: Data Analysis and Graphics With R, by Kabacoff, Robert I., Manning Pubns Co., 2015
-
Lecture Notes: BCP00
-
Grade: 成績 (updated at 06/24)
- News:
-
HW1:請寫一個程式模擬骰子丟100次的結果,把結果畫出來,並計算平均值。
-
HW2: data.frame 練習,資料須至少有5個欄位與10筆資料。並使用$來存取欄位資料,使用mean()計算某一欄位的平均值。
-
4/22 18:30-20:00 期中考,範圍:課本第1章到第6章,地點:綜合大樓Y308教室
-
HW3: 畫XY散佈圖-使用plot與ggplot函式。資料的X與Y均為常態分佈。
-
HW4: 分別以for迴圈與while迴圈計算1到1000間的3的倍數和。
-
6/3 晚上19:30 舉行小考,範圍:第9章流程控制與第10章迴圈。
-
6/17 18:30-20:00 期末考,地點:綜合大樓Y308
-
期末考與學期成績已公佈,有疑問者請於6/27上午0900-1200與助教聯絡,學期成績有疑問者,請於6/27上午0900-1200與我聯絡,逾時不後。
-
Machine Learning (for 資訊科技科第二專長學分班)
-
Course Time: Thu 18:20-21:00
-
Classroom: AT337
-
Course Outlines: 1.Introduction to Machine Learning; 2. K-Nearest Neighbors; 3. Naive Bayesian Classification; 4. Decision Trees and Random Forests; 5. Hidden Markov Models; 6. Support Vector Machines; 7. Neural Networks; 8. Clustering; 9. Improving Models and Data Extraction; 10. Deep Learning
-
Textbook: “初探機器學習 使用Python”, O’Reilly, 2017(碁峰) (Thoughtful Machine Learning with Python: A Test-Driven Approach 1st Edition -Matthew Kirk)
-
Reference Books:
-
“Introduction to Statistical Pattern Recognition” by Keinosuke Fukunaga, Academic Press, 2nd edition,1990.
-
“Neural Networks and Learning Machines” by Simon O. Haykin, Pearson Education, Inc., 3rd Edition, 2009.
-
“Artificial Intelligence” - Leonardo Araujo dos Santos
-
-
Lecture Notes: ML00, MathFoundation, kNN, ML02, DL
-
News:
-
HW1: Design the k nearest neighbor classifier ( kNN) for the WINE dataset if half of the data are used as the training set, and the other half is used for testing. Compare the classification accuracy of training data and testing data, and the computation time. Note: You can download the WINE dataset from [wine.data], its detail information is here. The WINE dataset is the results of a chemical analysis of wines grown in the same region in Italy but derived from three different cultivars. The analysis determined the quantities of 13 constituents found in each of the three types of wines. It is from http://archive.ics.uci.edu/ml/datasets/Wine. The deadline is 23th April.
-
HW2: Single layer perceptron for the WINE dataset.
-
作業繳交方式:內容為主要演算法與程式片斷,包含測試資料與結果,以及簡短的討論或結論等。請把書面報告電子檔,程式及相關測試資料先以Winzip或WinRAR壓縮,並以學號為其檔名,上傳到以下指定的FTP網站:IP:140.120.182.124 , Port: 1996, Username: mlstudent, Password: ml19
-
學期成績已公佈
-